Leading AI makers at odds over how to measure "responsible" AI
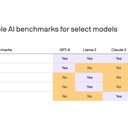
AI makers can't agree on how to test whether their models behave responsibly, per Stanford's latest AI Index, released Monday.
Why it matters: Businesses and individual users have little basis for comparison when choosing an AI provider to suit their needs and values.
Catch-up quick: "AI models behave very differently for different purposes," Nestor Maslej, editor of the 2024 AI Index from Stanford University's Institute for Human-Centered Artificial Intelligence (HAI), told Axios.
- But users lack simple options for comparing them, and there's no solution in sight.
- The most commonly used benchmark test for responsibility — TruthfulQA — is used by only three out of the five leading AI developers assessed by the Stanford team: OpenAI's GPT-4, Meta's Llama 2 and Anthropic's Claude 2 all use it, but not Google's Gemini or Mistral's 7B.
Developers' appetite for responsibility testing also varies widely.
- Meta gets top marks for benchmarking its Llama 2 model against three tests.
- Mistral's 7B model is not benchmarked against any of the five options tested by the Stanford team.
Today's benchmarks tend to specialize in narrow niches.
- TruthfulQA assesses whether a model gives honest answers based on its training data.
- RealToxicityPrompts and Toxic Gen look for propensity for hate speech.
Between the lines: "There's a clear lack of standardization, but we don't know why," HAI's Maslej told Axios.
- "Some of it could be cherry-picking," where developers test against the benchmarks that show them in the best light, or to make it harder for model users to identify a model's limitation, he suggested.
Zoom in: The lack of effective comparison tools helped inspire a Responsible AI Institute — supported by major companies — which released its own AI benchmarking tools.
The big picture: AI developers and academics are engaged in intense debate over which AI risks pose the most urgent dangers: immediate problems like discrimination through biased AI model outputs, or future "existential" threats from advanced AI systems.
By the numbers: Other key findings in the Stanford AI index include that U.S. organizations built a greater number of significant AI models (61) than those in the EU (21) or China (15).
- The U.S. led on private investment in AI, with $67.2 billion invested, but China led on patents, with 61% of all AI patents.
- In 2023, 108 newly released foundation models came from industry, but only 28 originated from academia.